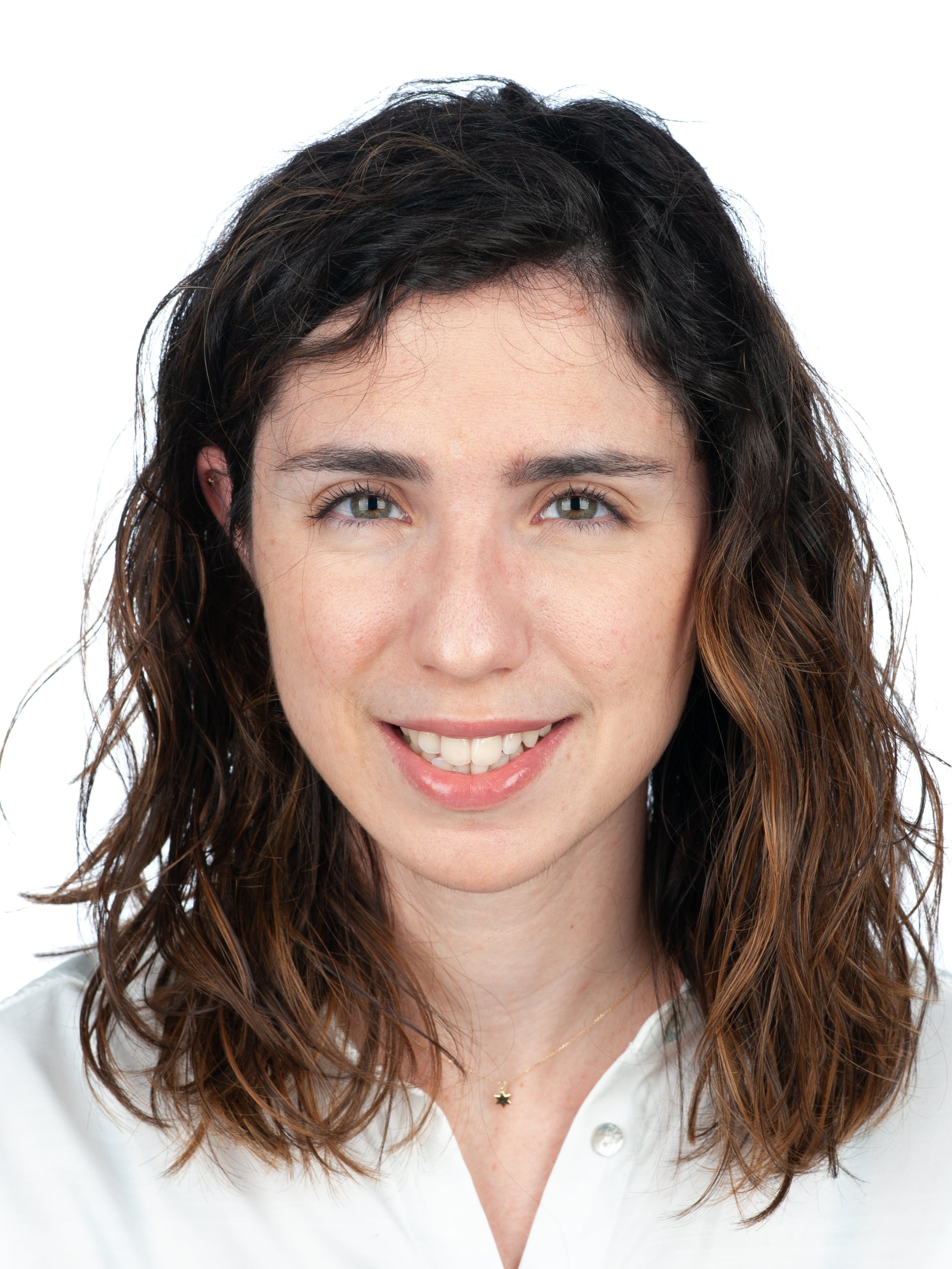
Postdoctoral researcher at the University of Zaragoza, applying physics-inspired machine learning to mechanical engineering problems. +4 years working with different supervised and unsupervised machine learning techniques, mainly applied to fluid dynamics and physical perception combined with computer vision methodologies. Teaching assistant in several courses, and engaged in academic and outreach communication, especially for children.
Education
PhD in Mechanical Engineering (February 2018–June 2022)
M.Sc. in Industrial Engineering (Sept 2015–June 2017)
B. Sc. in Mechanical Engineering (Sept 2011-June 2015)
Research stays
- École Polytechnique Fédérale de Lausanne (EPFL). From September 1st, 2021 to February 28th, 2022 (181 days). Advised by Prof. Jan Hesthaven.
Research areas
- Data-driven modeling and machine intelligence
- Physically-based simulation
- Model Order Reduction
- Digital and hybrid twins
Training
An ECCOMAS Advanced Online Course on Computational Structural Dynamics. Czech Academy of Sciences. September 2020.
The Art of Modeling in Computational Solid Mechanics. CISM – International Centre for Mechanical Sciences. October 2019.
Congress participation
Conference Talks
- Hybrid twins based on physically sound incremental learning. MMLDT-CSET 2021-Oral Communication San Diego, US; ONLINE.
- Deep learning of fluid dynamics from free surface data for full state reconstruction and correction. Eccomas Young Investigators Conference-Oral Communication Valencia, Spain; ONLINE.
- Physically sound deep learning development of digital twins from partial measurements of real-world data. Coupled Problems in Engineering 2021-Oral Communication Chia Laguna, Italy; ONLINE.
- Thermodynamics-based learning of fluid dynamics from partial information. Joint European Thermodynamics Conference 2021-Oral Communication Prague, Czech Republic; ONLINE.
- Hybrid twins for fluid applications. World Congress in Computational Mechanics -Oral Communication Paris, France; ONLINE.
- Manifold Learning of complex fluid behavior for real-time simulation. Eccomas Young Investigators Conference-Oral Communication Krakow, Poland.
- Data-driven learning of slosh dynamics. Congress on Numerical Methods in Engineering-Oral Communication Guimaraes, Portugal.
- Data-driven, reduced-order modeling and simulation of free-surface flows. Coupled problems in Engineering-Oral Communication Sitges, Spain.
- Data-based manifold learning of sloshing dynamics. DataBest2019-Oral Communication Paris, France.
Poster participation
C2D3 Virtual Symposium 2020. Cambridge, UK (On-line).Digital twins of fluid dynamics for realtime interaction.
Congress on Numerical Methods in Engineering 2019. Guimaraes (Portugal). Data-driven learning of slosh dynamics.
Publications
- Moya B., Pichi F., Hesthaven J., A convolutional graph neural network approach to model order reduction to non-linear parametrized PDEs. In preparation.
- Moya, B., Badías, A., González, D., Chinesta, F., & Cueto, E. (2022). Physics-informed Reinforcement Learning for Perception and Reasoning about Fluids. https://arxiv.org/pdf/2203.05775.pdf
- Moya, B., Badías, A., González, D., Chinesta, F., & Cueto, E. (2021). Physics perception in sloshing scenes with guaranteed thermodynamic consistency. IEEE Transactions in Pattern Analysis and Machine Intelligence. https://arxiv.org/abs/2106.13301
- Moya, B., Badías, A., Alfaro, I., Chinesta, F., & Cueto, E. (2020). Digital twins that learn and correct themselves. International Journal for Numerical Methods in Engineering. https://doi.org/10.1002/nme.6535
- Moya B, Alfaro I, Gonzalez D, Chinesta F, Cueto E (2020) Physically sound, self-learning digital twins for sloshing fluids . PLOS ONE 15(6): e0234569.https://doi.org/10.1371/journal.pone.0234569
- Moya, B., González, D., Alfaro, I., Chinesta, F., & Cueto, E. (2019). Learning slosh dynamics by means of data. Computational Mechanics, 64(2), 511-523. https://doi.org/10.1007/s00466-019-01705-3
Book Chapters
- Chinesta, F., Cueto, E., Grmela, M., Moya, B., & Pavelka, M. (2019). Learning Physics from Data: a Thermodynamic Interpretation. In: Barbaresco, F., Nielsen, F. (eds) Geometric Structures of Statistical Physics, Information Geometry, and Learning. SPIGL 2020. Springer Proceedings in Mathematics & Statistics, vol 361. Springer, Cham. https://doi.org/10.1007/978-3-030-77957-3_14
Datasets
Computational fluids. https://github.com/beatrizmoya/sloshingfluids
Real fluids. https://github.com/beatrizmoya/RLfluidperception
Outreach contributions
- Self-learning digital twins for sloshing fluids. https://www.youtube.com/watch?v=d1JyhPNkLkU
- Physics perception in sloshing scenes. https://www.youtube.com/watch?v=Qlb1VpWRVaQ
- Physics-informed Reinforcement Learning for perception and reasoning about fluids. https://www.youtube.com/watch?v=ikPgZMpsCFk
- Aprender a razonar, el ser o no ser de la robótica. Heraldo de Aragón. https://www.heraldo.es/noticias/aragon/2021/11/20/aprender-a-razonar-el-ser-o-no-ser-de-la-robotica-1534983.html
- European research night 2020: https://www.youtube.com/watch?v=Wu2KVFctSBI&list=PLbIY5lVtgXEIzAhD-y9Lm-IMMUCdAYCPb&index=14
- Radio interview 2020: https://www.ivoox.com/mananas-onda-aragonesa-el-gemelo-audios-mp3_rf_58255616_1.html
- Talks to children 2021: https://aragonuniversidad.es/universidad/cerca-de-800-alumnos-de-primaria-de-aragon-debaten-con-los-investigadores-como-sera-la-vida-en-2075/
- Podcast about digital twins: https://www.i3a.es/es/podcasts?field_categoria_podcast_value=divulga_i3a
- Una ingeniera en cada cole 2022. (A female engineer in each school)